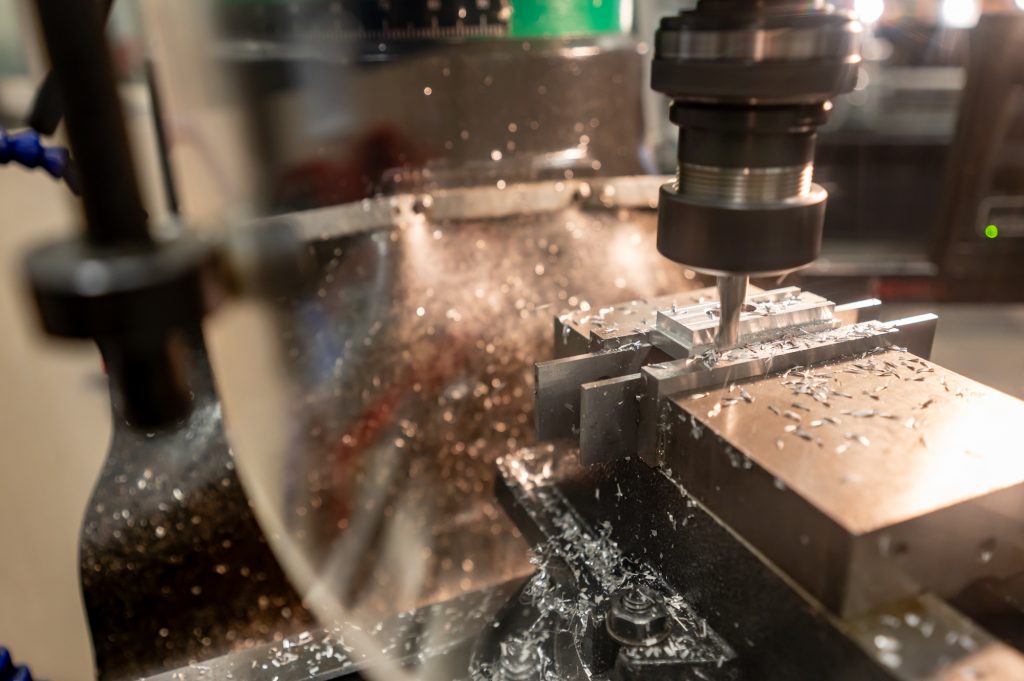
Challenge 1 – The Making challenge
To create new hybrid manufacturing processes, that combine multiple Additive Manufacturing (AM) process with precision machining and coating processes to create components that disrupt the traditional functional trade-offs of Size, Weight and Power (SWaP) through techniques such as varying the material properties within a part and harnessing the digital production of optical components.
Project M1: Complete Additive Manufacturing of a Laser Chassis
The joint team is delivering a program of application-specific research and development designed to de-risk the complete manufacturing of an optical laser assembly and chassis using laser-powder-bed fusion technology, and address major barriers currently perceived for the successful uptake of AM technology in future laser products.
The team is focussing on a future-generation hand-held laser target designator as example case, with learnings transferable to other platforms and systems. This example will benefit the most from volume and weight savings promised by AM, but it is critical to maintain or improve on laser system efficiency. An ultra-compact design will challenge the management of internally generated heat in the laser chassis, whilst ensuring the optical performance over a large temperature range. AM offers opportunities in the material choices, novel geometries and interface-engineering between optics and AM-printed parts, all of which are being developed and critically evaluated in this project.
This project is core to the Partnership Roadmap benefitting from the prior work of the team members and feeds into partnership projects, PhD topics, technology roadmaps and future major project proposals to external stakeholders.
Project S1: Novel AM materials to manage laser chassis thermal expansion
The objective of this project is to explore the use of novel materials and forms enabled by additive manufacturing (AM) to effectively manage thermal expansion and distortion in a laser chassis. Minimizing the degradation of optical performance due to thermal expansion during laser operation is a key goal. Although additive manufacturing has seen limited adoption in high-precision laser systems, it holds the potential to provide new solutions to this problem. We will focus on laser powder bed fusion (PBF) AM, the dominant metal AM process, which ensures compatibility with the existing supply chains. This project, along with long-term project M1, aims to contribute to the overall ambition of manufacturing a laser chassis by AM, future-proofing the design, and assessing the commercial advantages of utilizing new materials despite the additional cost associated with AM products.
Challenge 2 – The Manipulation Challenge
M2: Cyber-Physical Fabric for Robotic Assembly and Maintenance
A programme of application-specific research designed to explore the automated, cobotic and/or autonomous alignment of an optical laser assembly using robotics and mechatronics. Research will focus on automated alignment strategies and machine learning. The core project goals are to provide design and manufacture rules on automated laser assembly paired with a demonstrator shared with the M1 Additive Manufacturing system.
Challenge 3- The Computation Challenge
M3: Sensor Data and Resource Management for Autonomous Airborne Platforms
Previous projects (University Defence Research Collaboration (UDRC) and DASA) have demonstrated that sensor networks and multi-sensor fusion techniques address key challenges in intelligence, surveillance, target acquisition, and reconnaissance (ISTAR) but data-driven sensor tasking and resource management, particularly when considering autonomous platforms, has still not been adequately addressed. A sensor-systems Engineering approach is required which builds on an existing Leonardo-funded PhD studentship and aligns with the related NATO Set group work. The main objectives are to develop the necessary sensor processing, information fusion and resource management systems to provide air and surface surveillance with over the horizon situational awareness providing detection, tracking, and recognition capability of surface and airborne objects within sufficient timescales to react appropriately and to achieve this capability, necessary to develop state-of-the-art signal processing solutions for enhancing a multi-sensor radar system.
S2. Next-generation sensing using event-based processing and neuromorphic computing
A programme addressing the current limitations of traditional frame-based sensors and associated processing pipelines with a new family of algorithmic architectures that mimic more closely the behaviours of biological brains. In such architectures, referred to as spiking networks, the data is encoded via trains of spikes or events, which allows processing using simple elementary operations. They can offer increased processing speed and reduced power consumption, especially when implemented on dedicated hardware (neuromorphic chips or FPGAs). The main challenges associated with event-based processing, to be addressed are computationally efficient and reliable training of spiking architectures for imaging and sensing tasks. More efficient methods have recently been shown to lead to smaller architectures, i.e. lower-cost and lower-power computing units, while maintaining good information extraction performance. The project will address two distinct elements: Data Extraction: Using a combination of statistical and data-driven methods, we will develop methodological tools to extract information from streams of events associated with radio frequency (RF) and electro-optical (EO) sensors and learning for machine learning (ML): supervised offline learning as well as the emerging powerful self-supervised learning techniques that require little or no ground truth data and could lead to longer projects covering online learning (during deployment) as well as implementation on dedicated hardware.